Quick & Dirty A/B Testing Hacks for New Blogs
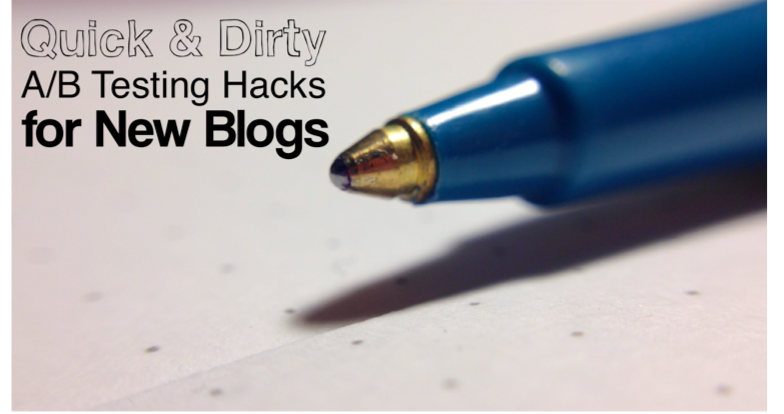
Today's guest post is written by Andrew Littlefield, an Atlanta resident working at a healthcare private practice who has a strong interest in content marketing, growth hacking, and tech startups. You know what I love about football?Statistical noise.There’s a lot of randomness in football. It’s played outdoors in all types of weather: from sweltering heat, to monsoon-like rain, to white-out blizzard conditions. Even the ball’s shape plays a role in the randomness of the game; it can bounce any which way. Then there’s the fact that NFL teams only play 16 games a year in their regular season. That all adds up to what’s known in the stats world as a small sample size.
Small sample sizes are the enemy of predictability.
All too often, the best football teams have their seasons derailed due to freak occurrences: a quarterback with the flu, an unlucky bounce, or a bad call.On the opposite end of the statistical spectrum is baseball. Major League Baseball teams play 162 games in a season. Players see hundreds of at-bats per year; thousands of pitches are thrown.This large sample size neutralizes the random spikes of statistical noise that occur throughout a season. A starting pitcher catching the flu and having a bad game means relatively little when stacked up against another 161 games.
Small Sample Sizes Injects Instability Into A/B Testing Results
Start-up marketers and growth hackers are always trumpeting the value of A/B testing, and for good reason. With limited budgets, start-ups must market with surgical precision. However, many start-up blogs are still in their early stages and haven’t built up a large audience just yet, which means their A/B tests may yield a small sample size of results.And just like with football, small sample sizes can make it hard to predict results and make inferences from them. So if you’ve just launched your blog or website and haven’t racked up large traffic numbers yet, how the hell are you supposed to run A/B tests with any kind of reliability?
Take a Trip Back to Psychology 101
So you only got 17 clicks on that A/B test you ran on some newly designed calls- to-action.How about you take a page from your Intro to Psychology textbook and measure your results twice?Small sample sizes are an issue frequently encountered in the field of psychology. Testing the effectiveness of treatments relies on taking data on a small number of subjects, so psychologists use a variety of research techniques to compensate for a lack of subjects. So why not use a few of these techniques with your A/B tests?
Multiple Baseline Design
In multiple baseline designs, the experimenter introduces the independent variable (the element we change to see if anything happens) across multiple settings. For the purposes of A/B testing on a website, we may use this design to test out new and improved copy on multiple pages and seeing if the results across each page are similar.The first step is to take baseline measurements. In our example we’ll set a goal of reducing bounce rate of a given page by optimizing certain design elements. Let’s say our baseline measurements give us a bounce rate of 41% across 50 page visits.We then introduce our independent variable (our page redesign) and measure bounce rate across 50 page visits again, revealing a bounce rate of 33%. Hey, great news! We’ve reduced our bounce rate. However, it’s a bit too early to claim success. There are too many possible confounding variables that might be skewing our results. So we run our experiment again on another, similar, page. Boom. Similar results again. We may decide to run this experiment a third or fourth time to ensure the effectiveness of our redesign, but we’re getting a pretty good idea that our experiment has been a success. The likelihood that another variable has caused our bounce rate to drop multiple times across multiple pages is low, and we can be pretty confident that our redesign was the cause for the reduction.
Withdrawal Design
In a withdrawal design (also known as an A-B-A design) a baseline measurement is taken, followed by the introduction of the independent variable, and finally (as you might have guessed) a withdrawal of the independent variable. If we see a change after introducing our independent variable, followed by a return to the baseline upon withdrawal of that variable, we can make a safe assumption that the change resulted from our intervention and not an outside cause.This design does present a roadblock: it relies on taking data on the same subject. This isn’t always an option when it comes to website visitors. So this design should be reserved for tests that involve lots of repeat visitors, like a blog or email list.Let’s run with that email list idea. We have a list of 50 email blog subscribers. Right now, their click-rate is hovering around 15%. We’re going to experiment with some personalization in the body of the email to see if we can raise that. First, we measure the click-rate across 10 daily blog subscription emails. As we thought, it’s right around 15%. Next, we introduce our personalization tactics for 10 emails. Suddenly, our click- rate rises up to around 21%. Finally, we return to baseline by removing our changes and going back to our original email format. Upon doing so, our click-rate drops down to around 16% across another 10 emails. At this point, we’ll want to reintroduce our email personalization, given the improved results. We could take data across the next 10 emails as well; just to make sure we see similar gains as our previous B section. 
Now’s the Time to Start Testing
A newly launched blog is naturally going to start small, which is the perfect time to start forming good testing and data tracking habits. Small sample sizes need not hold you back from taking a scientific approach to optimizing your site, it’s just a matter of having the right tools in your toolbox.